Imagine walking into a three-storied shopping mall with hundreds of shops and big screens displaying advertisements. What would you look for the most and what would you buy? Well, psychology has been playing an imminent role in designing advertisements and influencing our choice of products for a very long time now. Human attentional process is largely involved in determining which product or advertisement we are going to attend to the most. There is a reason why shops put up huge neon signs with flickering lights and bright colours. Or, advertisements of deodorant, cars and bikes project how beautiful women find such men using that particular product to be extremely attractive. In 1957, James Vicary, a market researcher, inserted the words “Eat Popcorn” and “Drink Coca-Cola” on the screen during an ongoing movie show.
The words were displayed for an extremely short period of time such that the audience could not consciously process the information, but long enough for their brain processes to perceive the information. It was found that during the intermission, sales of coca-cola increased by 18.1% and that of popcorn increased by 57.8%. What happened was that although the advertisement was not consciously attended by the audience, it was perceived subliminally and therefore influenced their choice. Subliminal messages have been later on used in hundreds of advertisements, targeted towards sex stereotypes and power perceptions. Take for example, Fig. 1 shows the advertisement of McDonald’s burger where a dollar sign appears at a particular point of time.
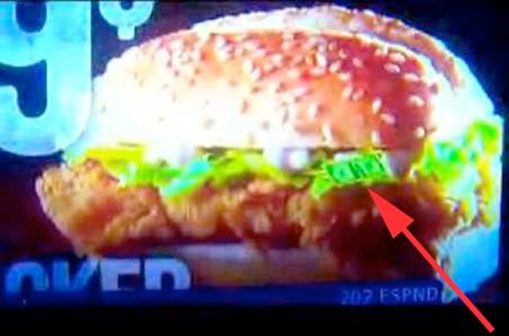
Fig. 1: McDonald’s advertisement showing a dollar bill
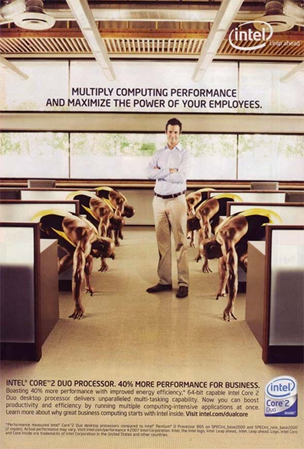
Fig 2. Intel advertisement
Or consider the advertisement of Intel (Fig. 2) where power is represented by the white man having authority over a number of black men. Both these messages in the advertisements are meant to trigger the need for power as in, the power that white men exerted on black men for centuries. Every day, we see hundreds of advertisements on television, social media and YouTube. So, how does such advertisement influence our choice of products? Which are the properties of a particular advertisement that contributes the most to influence our decision? It took psychologists, neuroscientists and marketing researchers several decades to realise how our brain processes advertisements and influence our choices. It was only during the last decade that Consumer Neuroscience as a field of academics and Neuromarketing as a commercial field of research emerged and gained lots of attention. Consumer Neuroscience lies at the centre of the overlapping boundaries of Psychology, Neuroscience and Marketing and intends to explain the science behind our decision to buy a product.
How does Consumer Neuroscience work?
Human brain has separate areas for decision making and experiencing emotions. It is primarily the function of the dorsal prefrontal cortex and orbito-frontal cortex of the frontal lobe to execute visual search, reason, judge and decide. While the amygdala, hypothalamus and nucleus accumbens located deep inside the brain gives the emotional colouring to an incoming stimulus. Deep inside the nucleus accumbens lies a “the pleasure centre” which determines “what” we like. Neuroscience facilitates recognizing the “why” behind such a choice. In 2011, Volkswagen produced an advertisement where a child was shown to attempt “The Force” on his parents’ washing machine, bikes and even on the family dog but nothing worked (Fig. 3).
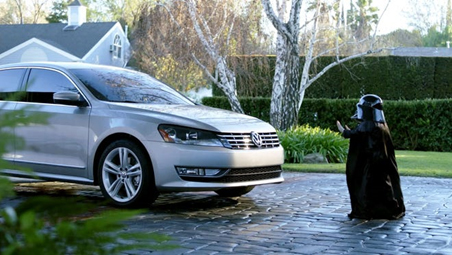
As his father arrived in Volkswagen Pissat, the boy went outside and tried to use “The Force” on the car for the last time. As the father realised what the boy was trying to do, he started the car’s ignition using the remote control and the boy was astonished, thinking that “The Force” actually worked. This advertisement scored the highest “neuro-engagement score” and won all the awards for that year including two Gold Lions at Cannes. When further investigated, research indicated that the advertisement engaged our attention and emotion to a large extent and therefore predicted end-market performance powerfully. Neuroscientists and psychologists working in this area, specifically work to predict which underlying attributes leads to higher attentional and emotional engagement. For instance, in a popular Indian advertisement, Katrina Kaif was seen biting and licking an Alphanso mango with the juice running down her chin. Sexual hints undoubtedly excite the “pleasure centre” of our brain and produce an overall positive impression of the product. Thus the Maaza advertisement sold the product after successfully manipulating our mindset.
Scientific evidence suggesting the role of brain areas in consumer decision-making is huge. For instance, brand preference over other brands is predicted by the activities of the ventromedial prefrontal cortex (vmPFC), dorsolateral prefrontal cortex (dlPFC), visual
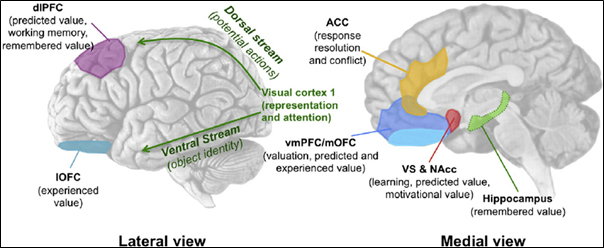
cortex, anterior cingulated cortex (ACC) and hippocampus (Deppe, Schwindt, Kugel, Plassmann, and Kenning, 2005; Deppe et al., 2005; McClure et al., 2004). As a matter of fact, all these brain areas are highly involved in recalling memories, emotional processing and decision making (Fig 4). For instance, in the infamous experiment on brand preference of Pepsi and Coca Cola, subjects were given a two tasting sessions one of which was blind. Researchers found that when they tasted Pepsi without knowing its’ brand, the “reward centre” of brain was activated more, indicating their preference. But when they were exposed to the brands, tasting Coca Cola stimulated hippocampus, mid brain and dorsolateral prefrontal cortex (areas for memory and emotion), an event that can be attributed to the nostalgia regarding its’ brand value. Undoubtedly, people like the brand of Coca Cola more than how it tastes.
Tricks of Consumer Neuroscience:
Consumer neuroscientists make use of different neuro-imaging techniques like Electroencephalograph (EEG), Functional Magnetic Resonance Imaging (fMRI) and Eye Tracking devices. fMRI techniques are more popular among these since it provides real-time information based on the oxygen level scanned at a particular brain area. Needless to say, research in Consumer Neuroscience is hugely expensive owing to the highly sophisticated techniques involved. Nonetheless, provided the benefits of predicting consumer choice based on neuroscience data, advertisement giants rarely hesitate to hire neuroscientists and psychologists to do the job. But that’s a commercial application of these techniques which are popular by the name of Neuromarketing.
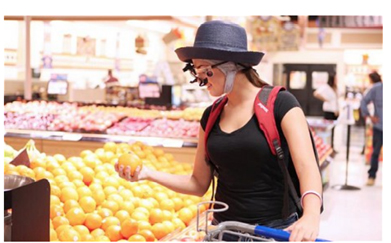
Fig 5. Shopper fitted with EEG equipment and Eye-tracker glasses (adapted from Ramsǿy, 2014)
For the sake of understanding and predicting consumer choice, neuroscientists rely more on EEG data and Eye-Tracking devices. In a typical experiment of Consumer Decision making, the volunteering customer is fitted with the EEG equipment and an eye-tracking device that is capable to track eye movements (Fig. 5). According to Steve Sands, a researcher at the Max Planc Institute of Lepzig, Germany, a single eye movement takes only 200 milliseconds and it is this 200 milliseconds within which a product can persuade us to buy it. In their study, Sands and others analysed 80,000 movements and found that 76% shoppers make in-store decisions for a purchase. Additionally, shoppers using cards have greater probability to make an impulsive decision (Ramsǿy, 2014).
Another paradigm of studying consumer behavior is by analysing images and videos in NeuroVision which is a Artificial Intelligence (AI) based computing software that predicts what our brain are most likely to see and miss. Instead of conclusions based on tracking eye movements, NeuroVision enables the researcher to predict which aspect of an image or product will be attended to automatically by the brain. Such prediction model is mostly based on image analysis and heat maps generated after analysis (Fig 6). Thus, visual attention these days can also be predicted using AI before even the shopper takes a look at the product!
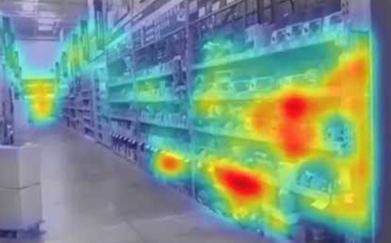
Fig 6. Heat map generated in NeuroVision
Miles to go:
Thanks to all the sophisticated brain imaging techniques, we now know that our brain makes a decision of making a purchase several seconds before we are making a conscious choice. However, even with the utilization of highly sophisticated techniques, predicting consumer behavior based on brain mapping, is still at its’ nascent phase. Most recent research in this area is focusing on predicting election polls and success of blockbusters based on brain mapping. However, the Neuromarketing industry is growing really fast and those days are not too far away when every aspect of advertising will be incorporating prediction models based on brain mapping and AI. Irrespective of the sales and profits facilitated by such prediction, it is indeed a fascinating feat for research in Psychology and Consumer Neuroscience that is contributing immensely to understand why we do what we do.
References:
- Plassmann, H. & Ramsøy, T. Z. & Milosavljevic, M. (2012). Branding the Brain – A Critical Review and Outlook. Journal of Consumer Psychology, 22, 18–36. 10.1016/j.jcps.2011.11.010.
- Deppe, M., Schwindt, W., Kramer, J., Kugel, H., Plassmann, H., Kenning, P., & Ringelstein, E. B. (2005). Evidence for a neural correlate of a framing effect: Bias-specific activity in the ventromedial prefrontal cortex during credibility judgments. Brain Research Bulletin, 67, 413–421.
- Deppe, M., Schwindt, W., Kugel, H., Plassmann, H., & Kenning, P. (2005). Nonlinear responses within the medial prefrontal cortex reveal when specific implicit information influences economic decision making. Journal of Neuroimaging, 15, 171–182.
- McClure, S. M., Li, J., Tomlin, D., Cypert, K. S., Montague, L. M., & Montague, P. R. (2004). Neural correlates of behavioral preference for culturally familiar drinks. Neuron, 44(2), 379–387.
- Ramsøy, T. Z. (2014). Selected Readings in Consumer Neuroscience & Neuromarketing. 2nd
- https://www.usatoday.com/story/money/business/2015/01/15/super-bowl-advertising-most-popular-ads-vw-volkswagen-the-force-budweiser/21761815/
- https://www.businessinsider.com/subliminal-ads-2011-5?IR=T#is-this-a-subliminal-racist-message-2
- https://www.businessinsider.com/subliminal-ads-2011-5?IR=T#a-hidden-dollar-in-this-kfc-sandwich-links-it-to-power-and-wealth-8
- https://www.youtube.com/watch?v=hQMh32WDSLI