The World Health organization has declared Covid-19 as a pandemic. As of April 29, an outbreak of Covid-19 has confirmed more than 3 million confirmed cases & more than 200,000 deaths worldwide. Various mathematical/statistical models have been developed to discuss the transmission dynamics. However, forecasting the future case in real time may not be accurate. We need to develop Artificial Intelligence (machine learning & deep learning) based mathematical/statistical models to overwhelm the constraint of epidemiological model approach.
Model Formulation:
Researchers follow few basic steps to construct any model. The steps are:
- Define: First we need to define the problem. The model selection depends on the amount of data availability. It may also refer to the difficulty of choosing the characteristic model from a large set of the computation model for decision making.
- Fit: Most essential part of the modeling. It captures the trajectory of the system with real time data.
- Predict: Predictive modeling is a method using machine learning technique to predict an outcome or event.
- Evaluate: Accuracy, precision & recall are three metrics to evaluate any model.
Last few weeks, we have observed various graphs/charts to predict the projected cases of Covid-19, but many of these models have been developed using data from previous research like SARS or MERS. Recently, researchers from MIT have developed a neural network using the data of Covid-19 to estimate the effective reproduction number. The effective reproduction number is one of the most important metric in epidemiology. It can be defined as “the average number of secondary cases per infectious case in a population made up of both susceptible and non-susceptible hosts.” The effective reproduction number is greater than one means epidemic has continued to spread exponentially & less than one indicates the point where we can flatten the curve & observe less infection.
Classical Epidemiological models predict the growth of disease, which groups total population into susceptible (S), exposed (E), infected (I) & recovered (R) populations. Recently, the SEIR model has extended by training a neural network to capture the transmission dynamics of Covid-19 [1]. The investigation relies on the surveillance data of Covid-19. The machine learning algorithm estimates the “infection plateau” for United States as somewhere between second-third week of April, 2020 (Figure 1). This projection of infected cases is similar to other predictions like Institute of Health metrics & Evaluation. The MIT model also suggests that quarantine policy & lock down policy are successful in getting the effective reproduction number less than one.
For healthcare system, it looks like promising news in terms of the number of confirmed cases, but it should not be considered as to start alleviating the control measures. Early termination of lock down could activate a catastrophic second wave with a sharper & rapid secondary peak.
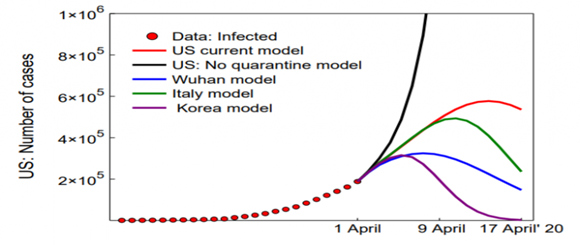
Figure 1: The figure depicts the model forecasting of confirmed infected cases in United States (Source: Reference [1]).
Reference:
- Dandekar, Raj, and George Barbastathis. “Quantifying the effect of quarantine control in Covid-19 .infectious spread using machine learning.” medRxiv(2020).
Visited 966 times, 1 Visit today