“Operations research (OR) is defined as the scientific process of transforming data into insights to making better decisions.”
–The Institute for Operations Research and the Management Sciences (INFORMS)
Introduction:
In the twenty-first century, especially in the last decade, the most trending domain of study is may be Data Science and Data Analytics. In this domain of study, people work with data from different fields and they use different tools and techniques from the domain of Mathematics, Statistics, and Computer Science to study and analyze the data. Then make some conclusion from the data and use them to predict the future of the phenomenon under study. Before the rise of data science as a domain of study, Operations Research analyst and Statisticians are used to do the similar kind of job. Due to these facts, the overlap between the domain of Data Science and the domain of OR is misunderstood. Also, there is a common perception that OR is not useful in for Data Science or Data Analytics. Actually, the marketing of OR products and services which are applied to solve the real world problems leads to this kind of misconception, as most of the time the end-users do not have an understanding or background of OR and data science. Another possible reason may be that the availability of machine learning models which are available as packages of several platforms like Python and do not really contain specific any OR models. In practical, OR tools and techniques are applicable to data science. In fact, a lot of ideas which are used in Artificial Intelligence (AI) and data science problem solving, have cross-pollinated from OR due to the large overlap in the techniques and methods used. In this blog, I try to explore these relations of OR with Data Science and Data Analytics.
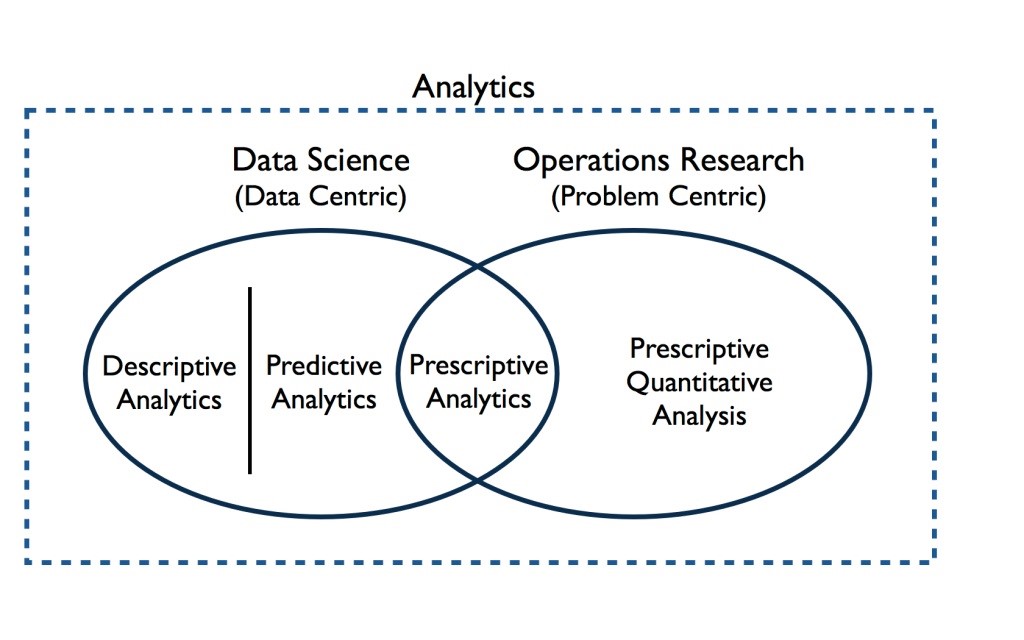
Operations Research and Data Science:
Before going to the discussion on the role and relation between Data Science and OR, let us try to understand another very important term called Analytics. According to INFORMS, Analytics is the application of scientific & mathematical methods to the study & analysis of problems involving complex systems. There are three distinct types of analytics:
i) Descriptive Analytics gives insight into past events, using historical data;
ii) Predictive Analytics provides insight on what will happen in the future; and
iii) Prescriptive Analytics helps with decision making by providing actionable advice [https://www.informs.org/Explore/Operations-Research-Analytics]. In an INFORMS podcast, depending on organizational backgrounds, Glenn Wegryn divides Analytics into two distinct camps: Data Centric Analytics where data is used to find interesting insights and information to predict or anticipate what might happen; and Decision Centric or Problem Centric Analytics which is used to understand the problem, then determine the specific methodologies and information needed to solve the specific problem. This data centric analytics are done by using Data Science whereas problem centric analytics are done by Operations Research. The above mention figure clearly give an idea about this. From the figure, it is very clear that there is a common point of interest from both the domain. Hence OR plays a very important role in Data Science domain.
Operations Research and Machine Learning:
Machine learning is the area of data science where most of the OR tools and techniques are used. Linear programming and Optimization techniques are fundamental part of the overall machine learning lifecycle. Some of the examples of OR are:
- Enabling smart human resource management by forecasting human resource requirements and optimizing daily schedule for resource persons (linear programming model)
- Increasing TV program viewership by optimal scheduling of programs’ promotion (linear programming model)
- Enabling supply chain transformation by providing AI/machine learning-based recommendations for optimized product utilization
- AI-enabled forecasting for retail and eCommerce applications to optimize funnel and customer traffic
- Data-driven optimization models for automated inventory management where we need to do warehouse management, inspection and quality control
Operations Research and Artificial Intelligence:
Another important area of data science is Artificial Intelligence where we can observe the use of OR algorithm. AI is used to build an automated system. Now, any real-life system have many decision variables and parameters, so if we want to build an automated system then we have to deal with a lot of decision variables. That’s why operations research algorithm must be a core engine in the system.
An Artificial Intelligence development lifecycle consists of the following steps: (Link)
Descriptive and Predictive steps:
- In the first step, we need to define the problem to be solved
- In the next step, we need to understand the current state of the problem and accordingly we have to define the work scope
- Next we need to develop a Machine Learning model, where the machine learning solution is developed and tested.
Prescriptive steps:
- Machine learning outputs or the predictions obtained using machine learning are given as OR inputs. Here, the OR techniques are used to make recommendations based on the outputs from the ML model. This is a critical step for the entire life cycle.
- Finally, the solution output is delivered to the client.
Covid-19 impact:
During the COVID-19 pandemic, more than ever, data science has become a powerful weapon in combating an infectious disease epidemic and arguably any future infectious disease epidemic. Computer scientists, data scientists, physicists and mathematicians have joined public health professionals and virologists to confront the largest pandemic in the century by capitalizing on the large-scale ‘big data’ generated and harnessed for combating the COVID-19 pandemic. (Zhang, Qingpeng, et al. “Data science approaches to confronting the COVID-19 pandemic: a narrative review.” Philosophical Transactions of the Royal Society A 380, No. 2214 (2022)). Covid-19 has a big impact on supply chain strategies also. People from data science community are analyzing “lessons learned” from the pandemic to better prepare and more efficiently and effectively respond to the next disaster, interested people can visit the following for a discussion on it (Link1).
Conclusion:
From the above discussion, it is very much clear that Data Science and Operations Research have some overlapping objectives with clear line of difference between these two domains of study. Also, we observe that there are several OR techniques and algorithms which have important role to play in different topics of data science. In my opinion, operations research together with data science and analytics is going to play a very important role to build the future of us.
Visited 1683 times, 4 Visits today