“The great paradox of automation is that the desire to eliminate human labor always generates new tasks for humans.”
Mary L. Gray
Introduction
The method of education is evolving regularly and dynamically. The delivery process in and out of the classroom has been changed to generate the targeted outcome. The regular involvement of technology in education helps to make it adaptive, more effective, and resourceful.
Machine Learning which is encompassing almost every aspect on earth, is also explored to find novel methods to enhance the dynamic change of the educational process. From cancer detection to predict financial data, driving a car to forecast weather, Machine Learning has left its footprint in almost everywhere. Adopting Machine Learning models helps educators to save their time in off-classroom jobs. For instance, a machine assistant can support an educator remotely for his students or scholars. The way of learning and progress of students is highly enhanced through the application of such methods. Teachers/educators gain insight into a student’s progression through the application of advanced Machine Learning algorithms and are very much help full to prepare an adaptive curriculum for the students.
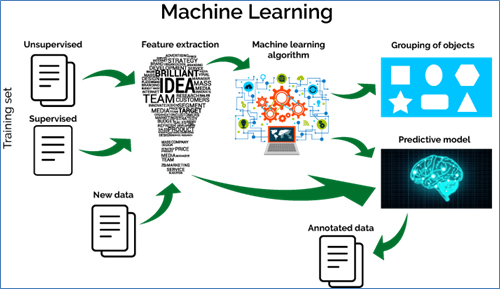
Figure 1
Image Source: https://towardsdatascience.com/workflow-of-a-machine-learning-project-ec1dba419b94
Machine Learning and its Types
The term Machine Learning is about writing self-learning programs with the help of appropriate mathematical models (process depicted in Figure – 1). It is the process of learning from EXPERIENCE which is nothing but the data supplied to it. One larger set of data is used to train (create knowledgebase) the machine and a smaller set of similar kinds of data are used to test its correctness to identify a similar type of new instance. This process of training and test continues until the machine achieves correctness near to 100%. Machine Learning is categorized into three main categories as in Figure – 2.
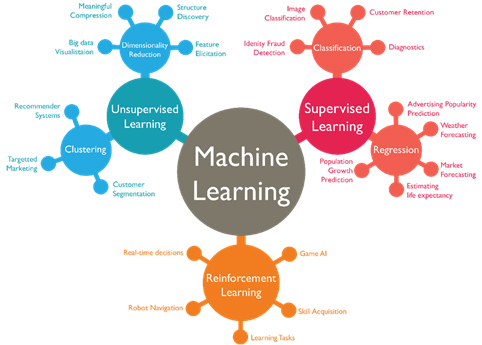
Figure – 2
Image Source: https://blogs.oracle.com/datascience/types-of-machine-learning-and-top-10-algorithms-everyone-should-know-v2
- Supervised Machine Learning: It is like a student is taught and evaluated by a teacher. The teaching process is similar to the training process and the evaluation process is similar to the testing process. In this method, a machine is a student, and data or dataset is the teacher. In this machine learning paradigm, a machine is also given scope to improve upon repeated training like a student.
- Unsupervised Machine Learning: It doesn’t require a teacher though; it is a categorization. If a machine is given a few types of objects, its job is to create categories comprises of those objects based on some common categorical features of objects.
- Reinforcement Learning: This method is an amalgamation of both the above methods. A machine may also learn from its mistakes. Whenever a machine makes a mistake it is notified and the algorithm is reinforced (re-iterated) to improve the performance. After a considerable amount of repeated learning, it makes the least mistake if the underlying algorithm is a robust one.
The Manifestation
According to the recent studies Machine Learning algorithm has been applied to education in a multi-dimensional approach. The aspects of the educational process where Machine Learning has made a direct impact are described below based on those studies:
- Personalization and Customization in Learning: Machine Learning algorithm is capable enough to identify the problem/doubt areas of individual students by interaction through a crafted questionnaire. The answers to those questions will be encountered by the appropriate Machine Learning algorithm to categorize the students and address the students with possible customized comprehensive content so that the students can clear doubts fully. This process may also generate a report to the teacher after categorization for better monitoring and preparing personalized content for the students. In this method, no students will neither be overlooked nor be left behind. EdTech and MagicBox learning systems are following this method. It will thus address student retention problems by identifying the students who are at risk zone while learning.
- Content Analysis: ML models are also able to analyze the content to be taught in the class to measure the quality with the fixed standards set by the institute using statistical learning. It also helps to find out the relationship between the content taught and the student’s intellect by suitable distance metrics and classification methods. Analysis of the content to be taught in compliance with student’s intellect is a tedious job for a faculty, which is reduced with the interference of Machine Learning to a large extent.
- Bias-less Grading: Use of Machine Learning reduces the human bias in grading a student. Machine Learning using Natural Language Processing implements the automatic grading system where the machine evaluates the answer of a student using the model answer. It also checks for the cheating or copying in the examination by plagiarism check with the help of supervised learning.
- Analysis of Student’s Performance: Machine Learning, with input data such as grading score, behavior, attendance, the scale of interest, completion of assignments, etc. can analyze the performance and help students to fill-up the learning gaps accordingly by predicting suitable measures. Machin Learning approach like Recursive Clustering technique can be applied for analysis and predicting student’s performance. Also, Neural networks can be used for such analysis and prediction.
- Automated Feedback: In education system feedback is an essential part of further improvement in the teaching-learning process. Machine Learning, after analyzing the filled-up data by students and teachers comes with the objective feedback by which the assessment will be easier for students and teachers. Thus, Machine Learning eradicates human prejudice to provide feedback.
- Career Prediction: During the learning period in an institute, a student generates different = types of data through marks achieved in the examination, achievement in a competition, presenting in conference, participating in extra-curricular and co-curricular activities, developing projects, etc. Machine Learning after considering these data as features can predict the career path in multiple options using a decisive algorithm (such as Decision Tree). It is a great benefit for a fresher who has difficulty in identifying the correct career path and Machine Learning has proved to gain better accuracy than human counseling.
The above-mentioned areas are not only the aspects where Machine Learning has been applied. The other aspects such as curriculum development, conduction of examination, preparation of schedule, development of lecture plan, searching proper content for a particular student are also been endorsed by the Machine Learning algorithm. Also, Machine Learning is used for testing and evaluating various educational software with the use of algorithms like Artificial Neural Network, Decision Trees, Genetic Algorithm, Bayesian Learning, etc.
Conclusion
Though I am concluding this blog, it is the beginning of a new era of education or learning process where knowledge is imparted in an optimized and intelligent manner. The use of Machine Learning is going to reshape the educational process into a highly personalized learning environment where students and teachers can identify and use their potentiality at the highest level. The application of Machine Learning in the educational process will not replace the human teaching method rather it improves and enhances the existing methods. It will simplify each task and leads to the effective completion for both the entity with the slow adoption of Machine Learning models. An ample amount of training and awareness programs are required to adopt this method of AI/ML-based teaching-learning. We hope to see a future where learning is no more considered as a tedious task.
Further Readings
Visited 1677 times, 1 Visit today